Seniors named semifinalists in NFL’s sports analytics competition
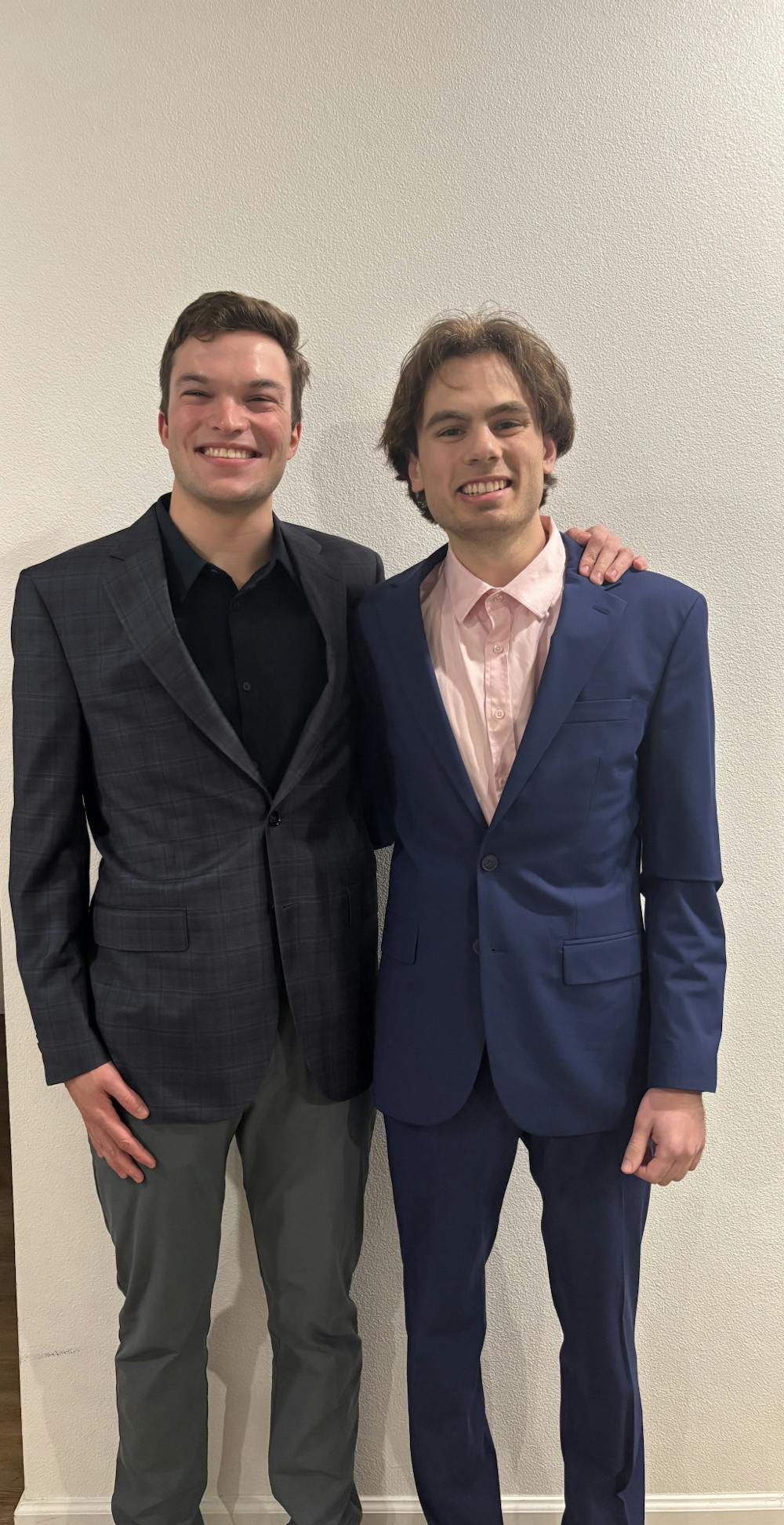
The NFL Big Data Bowl attracts thousands of participants annually. Sponsored by the NFL, the competition provides player tracking data and challenges participants to develop insights to inform real-world football decision-making. This year’s competition focused on pre-snap movement — how players move before the ball is snapped and its impact on gameplay. The competition took place virtually from Oct. 10, 2024 to Jan. 1, 2025.
Jonah Lubin and Charlie Wells, both Hanszen College seniors, were named semifinalists in this year’s competition. Lubin, who studies sport analytics and sport management with a concentration in sport law, and Wells, who studies computer science and math, worked together to analyze how pre-snap motion affects how open receivers are.
Their project sought to determine whether a receiver’s ability to get open was a result of skill or influenced by pre-snap movement.
“The objective was to quantify how much pre-snap movement helps receivers gain separation,” Lubin said. “We wanted to see if certain players were excelling on their own or if they were benefiting disproportionately from play design.”
Lubin and Wells analyzed player tracking data from the first eight weeks of the 2022 NFL season. This dataset, composed of millions of rows of data, provided movement information for every player on the field at every moment.
Lubin, who has experience in data science and football analytics, used machine learning models in R and RStudio, to predict receiver openness.
In particular, Lubin used gradient boosting techniques, which combine insights from multiple models to create a better prediction.
“Our model estimated a receiver’s openness by looking at their movement relative to defenders just before and after the snap,” Lubin said. “By isolating the impact of motion, we could see whether a receiver was actually skilled at getting open or if pre-snap movement was inflating their effectiveness.”
Wells handled data preparation and transformation using Python. His work included projecting player movements into the future and computing how far apart receivers and defenders were.
“Raw tracking data doesn’t immediately tell you which players benefited from motion,” Wells explained. “We had to build models that would predict where defenders would be if motion hadn’t occurred and then compare that to real-game scenarios.”
Initially, the team pursued a different hypothesis — predicting the likelihood that a player would receive the ball on a given play and the expected yardage gain. However, after testing, they determined the approach lacked statistical significance and refocused their efforts.
“We had already spent weeks on our first idea before realizing the data wasn’t supporting our hypothesis in a meaningful way,” Lubin said. “Shifting directions wasn’t easy, but ultimately, it was necessary to produce something insightful.”
After months of work, Lubin and Wells’ project placed in the top 10 out of thousands of submissions, earning them semifinalist recognition.
“It was rewarding to see our project recognized among some of the best in the competition,” Lubin said. “We spent so much time refining our approach that just making the semifinals felt like validation of the effort.”
While this was their first time competing, some of the researchers they competed against have been doing this competition for years. One of those researchers was Quang Nguyen, an experienced competitor known for his strong analytical approach and deep understanding of football data.
“Seeing our names listed alongside researchers from some of the top analytics programs and professionals in the industry was surreal,” Wells said. “It reinforced that the work we did was rigorous and meaningful.”
Their success drew attention from NFL professionals, Lubin said.
“I’ve received messages from people working in NFL analytics departments who were interested in what we did,” Lubin said. “It’s been encouraging to know that real decision-makers found value in our approach.”
Lubin and Wells said their complementary skill sets played a crucial role in their project’s success. Lubin focused on the football and statistical aspects, while Wells concentrated on data engineering and model implementation.
“We never had any major conflicts,” Lubin said. “Charlie has a strong technical background, and I have more experience in football analytics, so we each trusted the other to handle their part.”
Wells said he had similar feelings about combining their football and data analytics knowledge.
“Jonah understands the game at a deep level, and that helped ensure our analysis wasn’t just technically sound but also relevant,” Wells said. “There’s a big difference between running a model and actually producing something that makes sense in a football context.”
Beyond the competition, both Lubin and Wells saw this experience as a significant learning opportunity.
“It reinforced the importance of adaptability,” Lubin said. “If an idea isn’t working, you have to be willing to step back and rethink your approach.”
The NFL Big Data Bowl continues to be a major platform for aspiring analysts, providing opportunities to engage with real-world data and develop practical insights. For Lubin and Wells, the competition was not only an academic exercise but also an experience that sharpened their skills and connected them with industry professionals.
“This wasn’t just a school project,” Lubin said. “It was a real-world test of whether we could analyze data in a way that NFL teams would actually care about.”
Lubin plans to pursue a career in sports analytics, hoping to work with an NFL team.
“This competition reaffirmed my interest in football analytics,” Lubin said. “Ideally, I want to apply this kind of research in a professional setting.”
Wells, meanwhile, has accepted a position as a software engineer at Epic, a healthcare technology company.
“While I’m not going into sports analytics, this project showed me how much I enjoy tackling complex data problems,” he said. “That’s something I’ll take into my work in any field.”
For students interested in sports analytics, Lubin and Wells believe competitions like the Big Data Bowl are invaluable.
“If you want to work in this field, you need to get hands-on with data,” Lubin said.
As the sports analytics industry continues to grow, insights gained from projects like Lubin and Wells’ could shape how teams evaluate players and strategies.
“There’s no better way to learn than by diving in,” Wells said. “This experience pushed us and it gave us something meaningful to show for our efforts.”
More from The Rice Thresher
Sports Notebook: April 16-25
Rice men’s and women’s basketball programs each secured several transfer portal commitments last week.
04-23-2025 "Owl-American"
“Prepare for trouble and make it double!”
Men’s tennis wins AAC Championship with major upset
Rice men’s tennis traveled to Memphis, Tennessee, and pulled off a series of upset victories en route to winning the American Athletic Conference Championship.
Please note All comments are eligible for publication by The Rice Thresher.